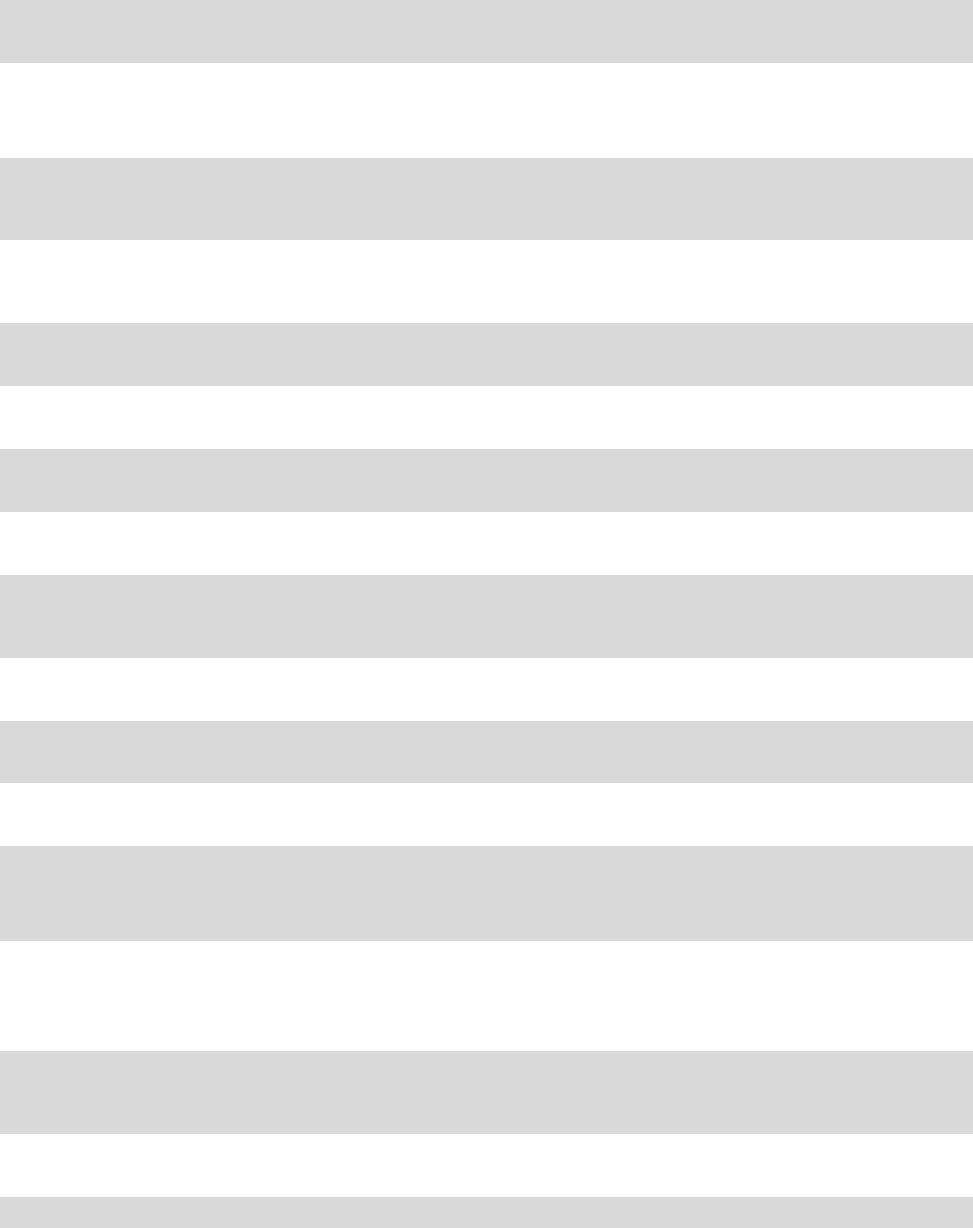
CONFIDENTIAL Page 172
Caroline Hoxby and Christopher Avery, “The Missing “One-Offs”: The Hidden Supply of High-
Achieving, Low-Income students,” Brookings Papers on Economic Activity 2013(1), pp. 1–65.
Christopher Avery and Jonathan Levin, “Early Admission at Selective Colleges,” Stanford
Institute for Economic Policy Research No. 08–31, March 2009, pp. 1–38.
David Card and Alan Krueger, “Would the Elimination of Affirmative Action Effect Highly
Qualified Minority Applicants? Evidence from California and Texas,” Industrial and Labor
Relations Review 58(3), 2005, pp. 416–434.
Daniel Koretz, Michael Russell, Chingwei David Shin, Cathy Horn, and Kelly Shasby, “Testing
and diversity in postsecondary education: The case of California,” Education Policy Analysis
Archives10(1), 2002, pp. 1–39.
Glenn Ellison and Parag Pathak, “The Efficiency of Race-Neutral Alternatives to Race-Based
Affirmative Action: Evidence from Chicago’s Exam Schools,” NBER Working Paper w22589,
2016, pp. 1–59.
Jimmy Chan and Erik Eyster, “Does Banning Affirmative Action Lower College Student
Quality?,” American Economic Review 93(3), 2003, pp. 858–872.
Julie J. Park and M. Kevin Eagan, “Who Goes Early? A Multi-Level Analysis of Enrolling via
Early Action and Early Decision Admissions,” Teachers College Record, 2011, pp. 2345–2373.
Mark Long, “Is There a ‘Workable’ Race-Neutral Alternative to Affirmative Action in College
Admissions?” Journal of Policy Analysis and Management 34(1), 2015, pp. 162–183.
Mark Long, “The Promise and Peril for Universities Using Correlates of Race in Admissions in
Response to the Grutter and Fisher Decisions,” ETS White Paper, 2015, pp. 1–35.
Peter Hinrichs, “The effects of affirmative action bans on college enrollment, educational
attainment, and the demographic composition of universities,” The Review of Economics and
Statistics 94(3), 2012, pp. 712–722.
Roland Fryer and Glenn Loury, “Affirmative Action and Its Mythology,” The Journal of
Economic Perspectives 19(3), 2005, pp. 147-162.
Roland Fryer, Glenn Loury, and Tolga Yuret, “An Economic Analysis of Color-Blind Affirmative
Action,” The Journal of Law, Economics, & Organization 24(2), 2007, pp. 319–355.
Sandra Black, Kalena Cortes, and Jane Lincove, “Apply Yourself: Racial and Ethnic Difference in
College Application,” NBER Working Paper #21368, 2015.
Sandra Black, Kalena Cortes, and Jane Lincove, “Academic Undermatching of High-Achieving
Minority Students: Evidence from Race-Neutral and Holistic Admissions Policies.” American
Economic Review: Papers & Proceedings 105(5), 2015, pp. 604–610.
Saul Geiser and Maria Veronica Santelices, “Validity of High-School Grades in Predicting
Student Success beyond the Freshman Year: High-School Record vs. Standardized Tests as
Indicators of Four-Year College Outcomes,” Research & Occasional Paper Series CSHE 6.07,
2007, pp. 1–35.
Sean Reardon, Rachel Baker, and Daniel Klasik, “Race, income, and enrollment patterns in highly
selective colleges, 1982-2004,” Center for Education Policy Analysis, Stanford University, 2012,
pp. 1–27.
Sharmila Choudhury, “Reassessing the Male-Female Wage Differential: A Fixed Effects
Approach,” Southern Economic Journal 60(2), 1993, pp. 327–340.